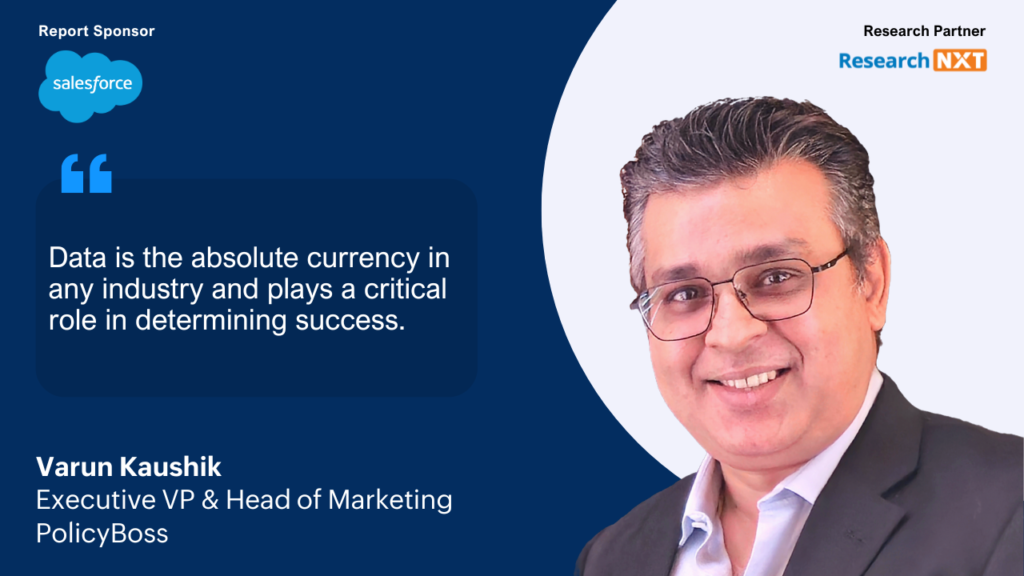
Revolutionising Insurance: How PolicyBoss is Leveraging AI to Redefine Distribution, Personalisation, and Customer Experience
PolicyBoss, once a leading B2C insurance broker, is now a force in the insuretech world. In this interview, Varun Kaushik, Executive Vice President and Head of Marketing, reveals how AI is fueling this transformation. From optimising data to personalising every customer interaction, Varun explains how PolicyBoss is leveraging AI to streamline operations, conquer industry challenges, and redefine the future of insurance distribution.
Key Highlights from the Interview
- Transformation through AI: PolicyBoss has successfully transitioned to a digital-first B2B2C model, leveraging AI for targeted marketing campaigns, predictive segmentation, and personalised user experiences through tools like chatbots and web customisation, resulting in higher engagement and conversion rates.
- Streamlined Marketing Processes: AI-powered tools have significantly reduced costs and time for creating marketing assets, enabling better customisation and improving campaign efficiency.
- Data Management and Privacy: Robust systems ensure real-time updates, data accuracy, and adherence to compliance standards, prioritising user privacy and preferences through a zero-spam policy and a focus on inbound marketing.
- Strategic and Scalable AI Implementation: Emphasis is placed on small-scale deployments and iterative learning to adapt AI solutions to existing workflows, ensuring a balanced cost-benefit analysis without requiring complete operational overhauls.
- Future of Insurance with AI: AI is driving innovation in personalised insurance offerings, underwriting, and claims processing, while regulatory advancements such as usage-based motor insurance point to a future of greater flexibility and customer-centric models.
“The difference between winning and losing often lies in how effectively a business interprets and acts on data insights. Intelligence—whether artificial or business-driven—is the foundation of success.”
Please share a brief overview of your journey at PolicyBoss, leading up to your current position as Executive Vice President and Head of Marketing.
Varun: At PolicyBoss, we’ve undergone a significant transformation since our early days as a brick-and-mortar insurance broker. Up until 2017-2018, our focus was primarily B2C. However, with the introduction of the IRDAI POSP (Point of Sale Person) guidelines, we pivoted to a B2B2C model. Today, 80-90% of our business is agent-driven, with over 85% conducted online through our proprietary digital platforms. This shift has been supported by robust advancements in our back-end systems, including LMS, CRM, automation, and RPA for reporting and analytics.
Our in-house platform has evolved into the backbone of our agency business, functioning as our LMS, CRM, ERP, and back-office management system. More recently, we’ve integrated generative AI to build a fully digital, zero-manual-intervention onboarding process and launched industry-first features like ‘Live Brokerage’ and ‘InstaPay,’ which have significantly boosted agent productivity and online policy volumes.
These innovations have not only reshaped our business model but also set new benchmarks in insurance distribution, creating a seamless, tech-driven experience for our agent community.
Can you share how your current role involves the implementation of AI within your organisation and at which stage of AI implementation your organisation currently stands?
Varun: For me, AI represents an evolution from rule-based automation to a more intelligent and humanised form of decision-making and problem-solving. At PolicyBoss, our journey into AI began after successfully implementing automation, allowing us to elevate our processes and experiences to the next level.
Here are some practical use cases of AI at PolicyBoss:
- AI-Generated Images for Marketing
- Cost Efficiency: Previously, single-use stock images could cost ₹15,000–20,000. By adopting AI image generation tools like Ideogram Turbo 2.0 and Flux (Pro, Fast Flux, and Ultra), we’ve reduced these costs drastically. Today, we pay a fraction of this cost with an annual subscription model.
- Time Savings: AI tools produce images that are 60-70% ready-to-use, minimising the need for post-processing like background removal. This has significantly reduced the time spent on image customisation.
- Learning Curve: Initially, generating the desired output was time-intensive, requiring detailed prompts and days of effort. However, with advancements in AI tools, we now achieve faster, more accurate results with minimal input.
- Generative AI in Outreach and Acquisition Pitches
- Dynamic Personalisation: Using generative AI, our acquisition pitches are conditioned to adapt dynamically based on the target audience. The AI engine refines and tones the messaging according to regional and demographic variations, eliminating the need for multiple pre-scripted versions.
- Improved Conversion Rates: AI tailors interactions for diverse personas, such as a South Indian gentleman in his 40s versus a North Indian gentleman in his 30s. This has enhanced the quality of conversions, yielding a 20-25% uplift in success rates during the initial months.
- Efficiency Gains: With AI handling pitch adjustments in real-time, we’ve reduced the time to market while improving overall productivity and engagement quality.
- Generative AI in Content Evolution
- Inspired by early AI use cases like Facebook’s “lookalike” targeting, we’ve applied similar principles to voice bots and chatbots. AI learns from initial scripts and user interactions to continuously refine and optimise pitches. This ensures relevance and contextual appropriateness for diverse audiences.
The adoption of AI at PolicyBoss has transformed how we approach marketing and outreach. While still a work in progress, the benefits are tangible—ranging from significant cost savings and improved productivity to enhanced personalisation, faster campaign roll-outs, quicker experience dissonance identification and higher-quality conversions. These early successes highlight AI’s potential as an enabler of smarter, faster, and more efficient marketing strategies.
Read More
You’ve transitioned from a B2C provider to an insuretech with a B2B2C model. In this transformation, what role did AI play? At which stages did AI contribute significantly, and to what extent is your business currently leveraging AI? Could you estimate the percentage or portion of your operations now driven by AI?
Varun: Our transformation journey from a B2C provider to a B2B2C model began with building a strong foundation of processes and workflows. AI entered the picture much later, as it requires a solid base to operate effectively. The focus initially was on digitising processes, integrating channels, and ensuring the readiness of systems for automation. Once that groundwork was laid, AI became a powerful tool for enhancing acquisition, engagement, and personalisation.
Here’s how we’ve integrated AI into our operations:
- Predictive Segmentation:
We use predictive segments to analyse user behaviour and create targeted campaigns. For example, based on the likelihood of a user converting, AI assigns them into categories like high likelihood, moderate likelihood, and unlikely. This segmentation allows us to craft personalised messaging for each group, optimising engagement. Over time, as campaigns run, the AI recalibrates these segments based on real-time responses, making subsequent outreach even more precise. This automated process improves targeting, reduces manual intervention, and enhances campaign efficiency. - Web Personalisation:
To attract career agents, we’re experimenting with creating a tailored, conversational landing page experience. When users click on an ad and land on our page, they will encounter a personalised welcome and an interactive walkthrough of the onboarding journey. This AI-driven approach will add a human touch to the digital experience, significantly improving engagement. Currently, we’re refining this concept by addressing language barriers and expanding beyond Hindi and English. - RFM Analysis:
For our existing user base, we employ Recency, Frequency, and Monetary (RFM) analysis to identify high-value users and those at risk of churning. This analysis highlights user interactions, such as how recently and frequently they engaged with our platform and their average transaction value. Based on these insights, we can design targeted retention and engagement campaigns to maximise user productivity and reduce churn. - Acquisition and Engagement Tools:
AI-powered tools like chatbots have transformed our acquisition strategy. We are also exploring dynamic content delivery on our platforms to provide a seamless and personalised experience for users at every touchpoint.
Through these implementations, AI has become an integral part of our operations. While still evolving, it has already enhanced productivity, improved targeting, and increased the quality of our engagements and conversions.
How critical is data management in building effective AI models and deriving meaningful insights? How do you ensure data is well-maintained and utilised effectively in your processes?
Varun: Data is the absolute currency in any industry and plays a critical role in determining success. The difference between winning and losing often lies in how effectively a business interprets and acts on data insights. While not everything is AI-driven, intelligence—whether artificial or business-driven—is the foundation of success.
For us, managing data is of paramount importance. We ensure our data remains current and accurate by employing the following strategies:
- Recency and Accuracy: We structure data based on its recency and the accuracy of interactions. This ensures that the information we act upon is both timely and relevant.
- Regular Refresh Cycles: Data fields are refreshed annually. If updates to data values occur, they are processed in real time to minimise any delays, ensuring our systems are always working with the most up-to-date information.
- Multi-Channel Utilisation: Our data supports various operational models, including call centres, branch networks, relationship management, remote support, and digital platforms. While each channel uses data differently, any updates are synchronised with the central repository in real time.
- Maker-Checker Process:
To ensure data enrichment and accuracy, a second level of manual verification is conducted at fixed monthly intervals. This maker-checker system adds an extra layer of assurance. - Streamlined Data Management:
By implementing idiot-proof processes for data handling, we ensure that our employees can work with data seamlessly. This low-error model of data enrichment enhances the overall effectiveness of our operations.
While data accuracy may not always reach 100%, these robust practices enable us to maximise the value of our data, driving better decision-making and outcomes across the organisation.
In your regulated industry, managing data privacy is critical. Has AI helped address these challenges, or do you see inherent complexities in implementing AI while ensuring data privacy?
Varun: We are rapidly transitioning into a permission-based marketing environment, where outreach will increasingly require prior consent from individuals. This shift isn’t far off and is driven by the current overload of information and unsolicited communication that has made many users resistant to unwanted messages. In a world saturated with spam and irrelevant messaging, there is a growing segment of users who have already taken control, opting to engage only with communications they deem necessary. This trend mirrors the high-net-worth individual (HNI) segment in banking, where customers expect tailored, permission-driven interactions.
AI will play a role, but it is just one of many tools required to navigate the complexities of data privacy. At PolicyBoss, we adopted a zero-spam policy several years ago, making a commitment to never send unnecessary messages or make unneeded calls to our users. We firmly believe the future of marketing, even in an outbound-heavy industry like insurance, is inbound. Our focus has shifted to creating tailored solutions for customers based on their specific needs, rather than bombarding them with generic outreach.
To support this, we’ve implemented comprehensive policies and practices around data privacy and user management. For instance, users have the ability to request updates to their personal data or even opt to have their data deleted entirely—except for information required by regulatory mandates. For example, while insurance sales records must be retained for seven years as per regulations, non-essential data, such as uploaded personal documents that didn’t lead to a sale, can be removed upon request.
This democratisation of data handling not only ensures compliance but also builds trust. By empowering users with control over their data and transparently communicating our privacy practices, we foster confidence and establish ourselves as a responsible brand. As data privacy becomes a cornerstone of customer trust, these measures are essential for staying ahead in an increasingly permission-driven marketing landscape.
In the BFSI industry, KYC is a key aspect of user authentication, requiring sensitive documents like PAN or Aadhaar. Managing this data responsibly is critical, as it comes with strict regulations on storage, access, and usage. As technology evolves, so will these rules, becoming either more restrictive or adaptable based on the landscape. With the rise of online fraud, regulators and brands must collaborate intelligently to ensure user safety while fostering trust.
It’s crucial for organisations to see data not just as numbers but as a reflection of real individuals. Respecting user privacy and choices is essential. A clear privacy policy and user-centric approach are essential for long-term trust. Missteps, like sending unwanted communications despite unsubscribe requests, can severely damage brand’s reputation. Instead, empathetic and transparent communication fosters goodwill and strengthens relationships.
What are some of the key challenges you have encountered during AI implementation? And what guardrails are you placing to prevent these known issues and mitigate associated risks?
Varun: To effectively integrate AI, it’s crucial to document your objectives and plan with clarity, avoiding overly abstract or emotional responses to outcomes. Approach AI deployment with a balanced perspective, acknowledging both its potential and the possibility of failure. Here’s a refined approach to implementing AI effectively:
- Plan and Assess: Begin by setting a baseline—identify your current situation and define clear objectives. For instance, in a marketing campaign, assess your acquisition run rate, costs, and areas where AI can add value, such as segmentation, communication, or asset creation. Treat this process like project planning, with defined milestones and expected outcomes.
- Start Small: Avoid going all in immediately. Start with 5-10% of your efforts or user base, and always maintain a control group outside the AI’s influence. This allows you to compare results and refine your approach based on reliable data. Gradual scaling minimises risk while providing actionable insights.
- Focus on Practicality: AI doesn’t require large teams of engineers or complex expertise. Equip your team with the tools and knowledge they need to use AI as a personal assistant, supplementing their existing skills. This democratises AI adoption and empowers even junior team members to contribute effectively.
- Continuous Learning: Dedicate time to reviewing and iterating on your AI use cases. Regularly gather your team to discuss what worked, what didn’t, and where automation or AI can improve processes further. This collaborative environment fosters innovation and ensures ongoing optimisation.
- Adapt and Empower: Encourage hands-on experimentation and learning within your team. As familiarity grows, individuals will naturally find ways to leverage AI more effectively. Even team members with no prior experience can become confident in using AI as they engage with it practically.
By methodically integrating AI and fostering a culture of experimentation and learning, organisations can unlock its potential while mitigating risks, ensuring a balanced and impactful implementation.
What emerging AI trends do you foresee influencing the insurance industry, particularly in marketing, over the next 3-5 years?
Varun: For a long time in India, insurance has been a highly generalised product category with limited scope for personalisation. However, AI is now transforming this landscape.
I recall a decade ago, while seeking an adventure sports cover, the options available were not only limited but also neither user-friendly nor affordable. The policies covered only a handful of sports and came with restrictive claim conditions. That scenario has significantly evolved, thanks to advancements in technology and AI.
Today, insurers can better understand consumer behaviour and preferences, using these insights to design more tailored product offerings. Regulatory initiatives like the sandbox model have further empowered insurers to experiment and innovate. A notable example is the introduction of usage-based motor insurance, where premiums are determined by kilometres driven, or the upcoming switch-on, switch-off models, allowing consumers to customise their coverage period.
AI is revolutionising key aspects of the insurance ecosystem—underwriting, claims processing, and policy purchasing—by simplifying the complexities traditionally associated with insurance. While actuarial science remains inherently intricate, the industry’s focus has shifted towards improving transparency and customer understanding. Insurers are leveraging AI to streamline explanations, enhance product clarity, and create easier consumer journeys.
This transformation not only makes insurance more accessible and relatable but also paves the way for increased penetration across the market. As AI continues to drive innovation, consumers can expect more choices, simplified products, and a seamless experience, ultimately fostering trust and adoption in the insurance sector.
We want to know your expectations from this report. What do you believe would be the most valuable data point or subject matter for you in this report?
Varun: When using any technology, including AI, it’s essential to have a well-defined value model. This involves clearly understanding the input costs, operational costs, and the expected output. Striking the right balance is crucial—don’t be overly cost-conscious and hesitate to proceed, but at the same time, avoid overspending without clear results. Financial prudence and strategic planning must go hand in hand.
When integrating AI into your processes, remember that the technology should adapt to your way of working, not the other way around. AI should blend seamlessly into your existing workflow, enhancing your efficiency and outcomes. Many fail by trying to reshape their methods to fit AI, which can lead to unnecessary complexity and poor adoption.
Set smaller, achievable milestones to measure progress effectively. AI delivers results that are typically faster and more measurable, allowing for easier assessment of success or failure. As with any initiative, attribution is critical. One should carefully evaluate the impact, cost, and degree of success relative to your goals.
By maintaining this balance and adopting a pragmatic, goal-oriented approach, you can harness AI as a powerful enabler without disrupting the core of how you operate.
This is still a practice in the making, and I look forward to what maturity and innovations drive AI adoption, particularly in a multi-channel and not omni-channel environment where disparity and diversity both are far higher. It would also be key to note how AI usage can be made cost-efficient at scale so that it enables revenue and augments experience building across use cases, across industries.